Confluent (CFLT) Q2 2023 Earnings Review
After delivering favorable results in Q1, Confluent continued on largely the same trajectory in Q2. Sequential growth rates even imply stabilization around 30% annually, after almost 2 years of deceleration from Covid highs. This growth is being led by rapid adoption of Confluent Cloud, with the licensed Confluent Platform offering for on-premise deployments demonstrating surprising resiliency as well.
The other factor providing support is a sharp improvement in profitability measures, with Non-GAAP operating margin increasing by 25 points year over year from -34% to -9%. The company expects operating margin to reach break-even by Q4. Free cash flow is following a similar path. Profitability was helped by an 8% headcount reduction in January, which notably doesn’t appear to have impacted growth.
Confluent’s recent revenue outperformance has been driven by customers exceeding their commitments for Cloud service usage. While customers may be limiting the size of future contractual obligations (reflected in RPO), engineering teams are choosing to allocate more spend to Confluent as the quarter proceeds. To me, this reflects the value customers are extracting from the Confluent Cloud product, as they willingly spend more than they had budgeted.
This may reflect the larger overall theme that enterprises are scrambling to improve their data processing infrastructure in anticipation of leveraging AI to create new offerings for their customers, employees and partners. While the expected benefits from AI are still unfolding and enterprises are largely in proof of concept mode, it is generally understood that effective AI requires good data.
Quality data as an input for AI and ML processing isn’t new, but the priority has been increased. This translates into more focus on consolidation, filtering, cleansing and categorization of silo’ed data stores. Data recency is also recognized as an advantage, which is creating a greater push towards real-time data distribution. It is this focus on reaching more data and delivering it in near real-time that is served by Confluent.
As other software infrastructure companies are experiencing rapid drops in NRR, Confluent appears to be holding up well. While they don’t report the actual values, management reported that overall NRR is still over 130% and for Cloud it exceeds 140%. These are pretty remarkable numbers in this IT environment for a product that is approaching an $800M annual run rate.
In this post, I review Confluent’s product strategy and discuss a few new announcements. Then, I will parse the Q2 results and discuss the trends that appear to be driving the recent outperformance in CFLT stock. For interested readers, I have published a couple of prior posts on Confluent, which provide more background on the product offering, competitive positioning and investment thesis.
Confluent's Product Strategy
Confluent sells a cloud-native, feature complete and fully managed service that provides development teams with all the tools needed to support real-time data streaming operations. The platform has Apache Kafka at the core, supplemented by Confluent’s additional capabilities to make Kafka easier to scale, manage, secure and extend. Some of these value-add features include visualization of data flows, data governance, role-based access control, audit logs, self-service capabilities, user interfaces and visual code generation tools.
Unlike some other forward-looking cloud infrastructure categories, like edge compute or even SASE, Confluent doesn’t have to convince enterprises of the value of data streaming. Over 75% of the Fortune 500 already use Apache Kafka at some level to accomplish this. Confluent’s task is to demonstrate that their data streaming platform, which offers many enhanced capabilities over self-managed open source Kafka, is worth the incremental cost.
While justifying an upgrade in the corporate data center represents a higher hurdle, Confluent Cloud provides enterprises with a managed solution on their hyperscaler of choice, eliminating the need to maintain a large team of operations engineers with Kafka expertise. Additionally, Confluent’s custom Kora engine delivers significantly higher performance, resiliency and storage efficiency than open source Kafka.
As part of the Investor Day presentation in June, Confluent management provided an updated view of the Confluent platform and its future revenue drivers. These have expanded beyond the core data streaming use case to encompass new capabilities that deliver all the capabilities necessary to power a modern data streaming platform. The top-level value-add modules include connectors, stream processing, stream governance and stream sharing.
Confluent could build a successful business just providing a cloud migration path from open source Kafka to Confluent. This would require just the Stream component of the platform to execute. However, leadership sees an opportunity to address a broader set of use cases as enterprises upgrade their data distribution infrastructure in preparation for AI workloads, to address privacy concerns and to facilitate data exchange with partners.
Beyond the potential for expanded scope, I find it interesting that a couple of these capabilities overlap with focus areas for modern data cloud platforms (previously called cloud data warehouses/lake/lakehouses) like Snowflake and Databricks. It seems that data infrastructure providers are converging on a similar set of use cases, as they scramble to become the primary provider of the consolidated data plane for the enterprise.
The cloud data platform vendors are extending from their base as the enterprise’s central data warehouse. Confluent is expanding to address similar use cases around data processing, governance and sharing from their foundation as a data streaming platform. I think this represents a logical progression for Confluent. Architecturally, I can see the efficiency in addressing some of these use cases directly in streaming workflows. Both approaches will exist in the enterprise, but these new capabilities would represent an incremental revenue source for Confluent.
Specifically, data distribution throughout the enterprise and externally with trusted partners requires strong capabilities in governance and controlled data sharing. As Confluent positions themselves to become the “central nervous system” or data fabric for the enterprise, it makes sense to extend this publish-subscribe pattern to include systems outside of the corporate network. Governance and sharing provide new capabilities that allow Confluent extend their reach in a safe and controlled way.
- Stream. At the core of Confluent’s offering is Apache Kafka, as the data streaming platform (DSP). The DSP allows companies to produce and consume (using the publish-subscribe pattern) real-time streams of data at any scale with strong guarantees for delivery. Today, this offering comprises the majority of Confluent’s Cloud revenue.
- Connect. To build a central nervous system for enterprises, a basic requirement is to connect to all systems involved. Confluent Cloud allows customers to run any Kafka connector in a cloud-native way, making them serverless, elastically scalable and fault tolerant. The Confluent team offers 120 connectors out-of-the-box to a variety of popular enterprise systems. There are also hundreds of open source connectors to less common systems available. In Q2, Confluent released their custom connectors offering, which enables customers to run any open source connector inside Confluent Cloud. This expands their reach beyond the basic set of connectors. Leadership reported that the on-premise, Confluent Platform product has approximately an 80% adoption rate for Connectors. This product is monetized.
- Process. Data processing is a key component of any major data platform. Stream processing extends basic data processing capabilities from SQL batches to full-scale data transformations with logic applied in real-time directly to the stream. Apache Flink is emerging as the standard for stream processing. Flink is available across a broad ecosystem of programming languages and interfaces. It is widely popular in the open source community and is used by some of the most technically sophisticated companies in the world, including Apple, Capital One, Netflix, Stripe and Uber. Every stream in Confluent likely will have some application code for data processing run outside of Confluent. The opportunity for Flink is to bring that development effort and processing logic within the stream on the Confluent platform, generating more consumption revenue. Leadership previously estimated this revenue source could grow to become as large as core streaming itself over time.
- Govern. When data moves between systems, teams or companies, governance is needed to ensure privacy and security of data. Streaming governance is Confluent’s fully managed governance suite that delivers a simple, self-service experience for customers to discover, trust and understand how data flows. Confluent has taken a freemium approach to stream governance, giving basic functionality to every customer. More recently, they have started to monetize with their Stream Governance Advanced offering. Management reports that two-thirds of their Confluent Cloud customers are using Stream Governance already. Revenue growth from Stream Governance Advanced is the highest of any product they have launched.
- Share. Inter-company sharing is a pattern the Confluent team noticed was gaining traction in the customer base in recent years. Customers in financial services and insurance needed to integrate to distribute key financial data streams within a complex network of publishers and consumers. Customers in travel needed to exchange real-time data on flights between airports, airlines, bookings companies and baggage handling companies. Retailers and manufacturers had to ingest real-time streams from suppliers to manage an end-to-end view of their inventory or supply chain. Oftentimes, these companies would have teams working out complex solution to facilitate this sharing, only to realize they were both distributing data internally through Kafka. Stream Sharing allows customers to enable cross-company data sharing within Confluent, but with strict access controls provided by Stream Governance.
These new capabilities can all be monetized separately. Confluent currently lists these as Value-add Components on their Pricing page for Confluent Cloud. Connectors and Stream Governance are in GA now and have pricing available. Stream Processing moved to early access with select customers in May. Stream Sharing was introduced in May with limited capabilities, but will continue to evolve into a full release with monetization.
Additionally, these capabilities are complementary and generate network effects for Confluent. They will both increase consumption within the platform and introduce Confluent to new potential customers. I have discussed in the past how Snowflake’s Data Sharing capabilities help them attract (and retain) new customers. I think the same argument for strong network effects can be made for Confluent with their new Stream Sharing capability.
"This means extending our central nervous system vision, something that spans the company to something that spans large portions of the digital economy. By doing this, the natural network effect of streaming, where streams attract apps, which in turn attract more streams is extended beyond a single company, helping to drive the acquisition of new customers, as well as the growth within existing customers. It’s essential to understand that these five capabilities: stream, connect, govern, process, share are not only additional things to sell, they are all part of a unified platform and the success of each drives additional success in the others. The connectors make it easier to get data streams into Kafka, which accelerates not just our core Kafka business, but also opens up more data for processing in Flink, adds to the set of streams governed by Stream Governance or they’re shareable by stream sharing. Applications built with Flink drive use of connectors for data acquisition and read and write their inputs from Kafka. Governance and sharing add to the value proposition for each stream added to the DSP. Each of these capabilities strengthens the other four." Confluent Q2 2023 Earnings Call
To calculate their market opportunity (TAM), the Confluent team used a bottoms-up approach. They examined their three customer segments, calculated the target population for each and then assigned an average ARR estimate. Their spend thresholds may skew on the high side for each cohort, but they have already hit these targets with some customers. As part of their Investor Day in June, management mentioned having 3 customers with $10M+ in ARR customers and 9 with $5M+.
When it was calculated in 2022, this exercise generated a $60B market opportunity. Using Gartner market data, management further predict a CAGR of 19% from 2022 to 2025. This yields a target addressable market of $100B by 2025, of which Confluent is currently less than 1% penetrated.
It’s worth mentioning that Confluent perhaps has a more favorable position to capture their share of TAM than some of the other software infrastructure categories that I follow. For example, observability vendors estimate a roughly similar sized TAM, but have to split that among several publicly traded independent providers (Datadog, Dynatrace, Elastic, Splunk, etc.). Security’s TAM is a couple times larger, but has many, many more vendors.
As compared to these, Confluent is the only independent, publicly traded provider of a data streaming platform. There are a few smaller private companies, which seem to have varying degrees of traction (Red Panda, Pulsar). The hyperscalers also offer managed Kafka and forms of pubsub systems, but none is as comprehensive as the Confluent platform.
Future Opportunities
Beyond the well understood path of converting traditional Kafka customer deployments to Confluent, there is a more disruptive aspect to Confluent’s strategy. That has to do with the logical combination of real-time streaming and basic stream processing capabilities. In many cases, skipping a central repository like a data warehouse makes sense as demand for near real-time updates increases.
During the product section of Investor Day in June, management walked through a number of usage scenarios for the Confluent Platform. It struck me that few of these involved sourcing or passing data through a data warehouse. For many use cases, it is faster to distribute data directly between operational sources and destinations. The major new capability to facilitate this direct pass-through is the addition of Stream Processing (through the Immerok acquisition based on Apache Flink). With this, sophisticated data aggregations and transformations can be addressed within the data streaming platform.
Granted, data will likely end up in a central repository eventually, like a data warehouse/lake/lakehouse for permanent storage, but a lot of the pre/post processing may be addressed within Confluent. This would shift a share of compute consumption from the cloud data platform providers to data streaming platforms like Confluent.
Confluent management has estimated that revenue from stream processing could eventually equal that for core data streaming. Viewed through this lens of facilitating real-time data distribution, that argument makes sense. Additionally, other new capabilities on the Confluent platform, like stream governance and data sharing mirror those of modern data platforms.
These use cases could peel off some cloud consumption revenue from the traditional cloud data platforms. As near real-time data distribution becomes the default, why not facilitate some portion of data processing and sharing through the streaming platform given that adequate governance is in place? I think this represents a reasonable argument for circumventing some portion of data sharing pipelines that would ordinarily be sourced from a consolidated data platform.
Q2 Earnings Results
Confluent reported Q2 earnings on August 2nd. As with the Q1 report, the post earnings response was positive with the stock popping by 16.2% the following day. Interestingly, this was the same jump experienced after the Q1 results. In both cases, the market discounted the stock significantly in the period before earnings. For Q2, it was down 21% from a recent peak just 2 weeks prior. This pre-earnings drop of about the same magnitude occurred in the two week period before Q1 earnings as well.
_CFLT Stock Chart (_Source – Koyfin. Click on the link for a reader discount)
Since Q2 earnings, CFLT stock has given back about 10% of its post earnings jump, but is still up about 45% YTD. Some of this coincides with a general AI stock sell-off that has occurred in August. Compared to other software infrastructure companies, Confluent’s valuation appears inline. The trailing P/S ratio is about 13.6 for a company growing revenue at 36%. Confluent remains unprofitable, however. The inflection to profitability and positive FCF margin within 6-12 months may provide further support for the valuation.
Revenue
Analysts were expecting revenue of $182.4M, representing growth of 30.9% annually and 4.6% sequentially from the Q1 result. This was at the middle of the range for $181M – $183M guided by the company. In Q2, actual revenue delivered was $189.3M, which was up 35.8% annually and 8.4% sequentially. Annualizing the sequential result reflects a 38.1% run rate, which is slightly higher than the revenue growth just delivered.
Subscription revenue grew even faster at 38.9% y/y to $176.5M and accounted for 93% of total revenue. In Q1, subscription revenue was $160.6M, meaning that subscription revenue grew sequentially by 9.9%. Annualized, that yields nearly 46% growth. Subscription revenue represents the core of the company’s growth as it is directly tied to demand for Confluent’s product offerings.
Services includes consulting work, implementation assistance and training. These are lower margin offerings necessary to help some customers with their transition to Confluent. Subscription revenue is more indicative of demand for Confluent’s core product offering and the higher growth rate here is a benefit.
Looking back over the past 2 years, investors will note that annual revenue growth has been rapidly decelerating from over 70% in Q4 2021 to 36% most recently. However, Q4 2021 represented a peak for the year 2021, with Q1 2021 revenue growth starting at 51%. More importantly, the amount of deceleration from Q1 to Q2 2023 was the smallest over that period at 240 bps. Further, the annualized sequential growth rate for Q2 would imply we are close to the bottom.
Revenue growth for Confluent Cloud is much higher than the overall company growth rate. The contribution mix is rapidly shifting towards Confluent Cloud with that product contributing 44% of total revenue in Q2, which was up 10% year/year from 34% of total in Q2 2022. Meanwhile, Confluent Platform’s contribution is decreasing, dipping below 50% for the first time in Q2.
Confluent Platform is growing more slowly, increasing by 16% annually in Q2 to reach $92.9M. This result exceeded the company’s expectations, however. In Q1, Confluent Platform revenue was $86.9M, also up 16% y/y. Most impressive is the 6.9% sequential growth rate in Confluent Platform, which implies that growth of Platform is actually accelerating. This was the second quarter in a row in which Confluent Platform growth outperformed management’s expectations. They attributed this to strength in regulated industries such as financial services and the public sector.
These entities are exhibiting a high demand for on-premise data streaming services, while they take longer to plan their cloud migrations. I find this interesting and may reflect a shift in priority for data streaming among engineering teams. One would think that a change to real-time data distribution would be saved for the cloud migration, but these organizations consider it important enough to justify ramping up within their on-premise installation. As I discussed earlier, I think a desire to prepare data infrastructure for AI is a contributing factor here.
Confluent Cloud revenue, on the other hand, grew by 78% in Q2 annually and 13.6% sequentially. Confluent added $9.9M of Cloud revenue in Q2, beating their guidance from Q1 for incremental Cloud revenue of $7.5M – $8.0M by a healthy margin of about $2.2M at the midpoint. The outperformance was driven by higher than expected consumption by some larger customers. Additionally, management noted that cloud as a percentage of new ACV bookings exceeded 50% for the seventh consecutive quarter.
The outperformance on Cloud represents an interesting signal. Customers appear to be conservative with their contractual commitments in advance (reflected in RPO), and then consume more resources (captured in revenue) within the quarter. While many software infrastructure companies are reporting customers optimizing consumption lower than expected, Confluent Cloud seems to be experiencing the opposite. Some large customers appear comfortable over-spending on Confluent Cloud.
RPO was $791.4M, up 34% y/y and 6.6% sequentially. In Q1, RPO growth was 35% y/y, so annual growth decelerated slightly, but sequential growth picked up. Current RPO, which represents RPO expected to be recognized within the next 12 months, was $514.8M in Q2. This was up 41% y/y and increased 7.9% from the $477M reported in Q1.
Management pointed out that the RPO growth rates were impacted by customers scrutinizing their budgets in the current spending environment. They again reinforced the observation that while commitments are lower, customers are actually consuming more than these commitments in the actual period usage. This provides a strong indicator of Confluent’s value to the organization, if management is willing to go over budget on Confluent consumption. With all of the attention being paid to spending trajectory, it is unlikely this over consumption is accidental.
"Our growth rates in RPO, while healthy, were impacted by a continuation of lower average deal sizes, a result of customer scrutinizing their budgets in the current environment. Despite the budget scrutiny, we remain encouraged that customers continue to derive value from using Confluent and consume more than their commitments, which is reflected in our revenue, but not in our RPO results." Confluent Q2 2023 Earnings Call
Confluent’s mix of revenue outside of the U.S. continues to grow, reaching 40% of total revenue in Q2. International revenue grew much faster than in the U.S., logging 45% annual growth versus 30% for the domestic business. This compares to 49% growth internationally in Q1 and 32% in the U.S. Lest we be concerned about the lagging growth in the U.S., Q2 domestic revenue increased by 9.6% sequentially, versus 7.1% for the international business.
Additionally, improvement in FX (neutral to weaker U.S. Dollar) should provide a little tailwind for international growth. While Confluent does not perform a constant currency calculation (because it charges in U.S. dollars), international customers would experience higher prices as the Dollar appreciates. When the opposite effect happens, Confluent prices feel lower for international customers. Other software infrastructure providers have reported a similar effect (Datadog, Cloudflare, etc.) and anticipate a small tailwind as FX improves.
Looking forward, management expects revenue for Q3 in a range of $193.5M to $195.5M, representing growth of 28.2% annually and 2.7% sequentially. This squeaked by the analyst estimate for $193.6M. The preliminary sequential guidance of 2.7% is lower than the 4.4% sequential growth projected from Q1 to Q2. That turned out to be 8.4% sequential growth in the Q2 actual, implying that Q3 sequential growth could decelerate into the high 6% range.
For cloud revenue, they expect $92.2M, which would be up 62% y/y and contribute 47.4% of total revenue. Given that they just delivered $83.6M of Cloud revenue in Q2, this represents an increase of $8.6M or 10.3% sequentially. This compares to the Q2 estimate in a range of $7.5M – $8M, which turned out to be $9.9M of incremental growth or a beat of $2.2M. Applying the same beat to the Q3 estimate would generate $10.8M of incremental growth and 12.9% sequentially. While that is a nice sequential growth, it is a bit lower than the 13.6% sequential growth just delivered. If we annualize the 12.9% sequential growth it equals the 62% annual growth projected, implying the deceleration in Confluent Cloud annual growth may be stabilizing.
For the full year, management raised the target revenue range by $7M to $767M-$772M for 31.4% annual growth. If Cloud represents 47% of total revenue in Q3, they expect that to increase to 48% – 50% in Q4. The slower increase in mix is attributable to continued strength in Confluent Platform growth. It should be noted that the $7M raise to annual revenue was equal to the beat on Q2 revenue. Management isn’t making any further assumptions about outperformance through the remainder of the year, given uncertainty about the macro environment.
I thought the Q2 revenue performance was strong and the forward guidance was okay. Analysts asked about the slightly softer raise to incremental Q3 Cloud revenue, given that the estimate is lower than what was delivered in Q1. I expect them to beat this target as they did in Q1. Given the remaining uncertainties in the IT spending environment, I think management is being suitably conservative.
Profitability
Confluent management has brought a sharp focus to profitability improvements over the past year. This has manifested most clearly with a roughly 24 point increase in Non-GAAP operating margin year/year. Prior to Q2, they had improved operating margin from -41.0% to -23.1% in the period from Q1 2022 to Q1 2023, representing a 1790 bps increase.
For Q2, they set a target of -16%, representing another 700 bps of improvement. This would generate ($0.08) to ($0.06) in Non-GAAP EPS. The actual results were much better with -9.2% operating margin. This beat their Q1 target by a whopping 680 bps and represented a 24.3 point improvement over Q2 2022. This result flowed through to Non-GAAP EPS which reached break-even at $0.00, beating the mid-point of guidance by $0.07.
Most of the improvement in year/year operating leverage came from efficiencies in the GTM effort. As a percentage of revenue on a Non-GAAP basis, S&M consumed 49% of revenue in Q2 2023, versus 62% a year prior, decreasing by 13 points. R&D decreased less, by 4 points and G&A by 2 points.
Investors will recall that Confluent announced an 8% staff reduction in January 2023. While a layoff is generally a disruptive event, the company has maintained its projected revenue growth in the two quarters following. This is actually a pretty bullish signal, as the period following the layoff would represent a distraction for most staff members. The positive financial objective of Confluent’s 8% workforce reduction was improvement in the path to profitability by a year.
Another contributor to the year/year improvement in profitability was a marked increase in gross margin. Total Non-GAAP gross margin improved 440 bps between Q2 2022 and Q2 2023, reaching 75.0% in the most recent report. For subscription revenue, the gross margin increases to 79.1%. Even on a GAAP basis, gross margin improved by 490 bps year/year. The improvement in gross margin was driven by two factors – healthy margins for Confluent Platform (which is license based) and lower cloud hosting costs on the hyperscalers through higher scale (volume discounts) and usage optimization.
It is worth noting that on a GAAP basis, the operating margin improvement was of the same magnitude, but starting at a more negative base. GAAP operating margin increased from -84.1% in Q2 2022 to -63.1% in Q2 2023, representing an improvement of 21.0 points. GAAP net loss per share stepped up from ($0.42) to ($0.35). Stock based compensation expense increased by 33.8% y/y from $68.9M to $92.2M.
Looking at cash flow, FCF was -$35.2M in Q2, which represented a slight improvement of $1.7M y/y. However, as a percentage of revenue, we have -26.5% a year ago and -18.6% in Q2. This shows about 8 points of improvement. Management had indicated that FCF margins should follow the trajectory of operating margins over time. On the earnings call, they stipulated that FCF margin should reach break-even roughly on a similar timeline to operating margin. That inflection is targeted for Q4 2023, which is now just two quarters away.
Looking forward, management projects -10% non-GAAP operating margin for Q3. This is below the -9.2% just achieved, but higher than the -16% target originally set for Q2. This implies that actual Q3 operating margin could hit the low single digits. Management took a similar approach with Non-GAAP income per share. For Q3, they set the target for ($0.01) to $0.00, which is just below the $0.00 delivered in Q2, but higher than the ($0.07) target originally set for Q2. With a similar beat, this will go positive in the Q3 actual.
For the full year, the improvement is more noticeable. Coming out of Q1, they raised the Non-GAAP operating margin target for fully year 2023 to a range of -14% to -13%. In Q2, they further increased the full year target to -10%. For income per share, they raised the target range from ($0.20) to ($0.14) in Q1 to $(0.05) to $(0.02) in Q2. These represent significant increases in my opinion, even more impressive than revenue growth in this environment.
Finally, the CFO provided long term targets for profitability. While revenue growth is maintained at 30% or higher, they expect to deliver operating margin in the 5-10% range. FCF margin would be inline with this as well. The long term trajectory would bring both operating and FCF margin to 25%+. This compares well to targets shared by other software infrastructure companies with a similar product mix.
These long term targets are slightly higher than those presented at the Investor Session in May. The long-term gross margin target previously was 72%-75% (now 75%+. The long-term operating margin target was 20%-25% and is now 25%+.
Customer Activity
Confluent demonstrated strong customer engagement in Q2, primarily with their larger spenders. The big opportunity for the company is to increase the annual commitment from their largest customers, as it doesn’t take many $1M plus customers to keep driving durable growth at their current revenue scale.
Of the reported customer metrics, Confluent experienced the highest growth in $1M+ ARR customers, which increased by 48% y/y. For Q2, they added 12 of these. That followed additions of 8, 15 and 13 in the prior three quarters. Confluent added 11 $1M+ customers between Q1 and Q2 2022, so this most recent Q2 outperformed slightly on an absolute basis.
Growth of $100k+ customers was healthy as well, with 69 added in Q2. This represented a 33% annual increase and 6.4% sequentially. Prior quarters registered counts of 60, 70 and 83, so roughly holding in the same absolute range. Q2 of 2022 added 59 of these sized customers, meaning this Q2 represented a healthy jump.
The total customer counts are growing more slowly. For Q2, Confluent added 140 total customers, up 17% y/y and 3.0% sequentially. This rate is on the lower end of prior quarters, which increased by 160, 290 and 120. A year ago in Q2 2022, Confluent added 0 customers sequentially, which was a consequence of a change to the sign-up process for the Cloud product.
As investors will recall, Confluent previously required any new Cloud customer to enter credit card information and begin incurring charges for any usage. After Q1 2022, new Cloud customers could get immediate access to the service for limited usage. This is called the Basic plan and allows for $400 of credits before requiring a credit card payment.
Once they are ready to ramp up, then customers switch to the Standard plan which is paid. I suspect this has created a lag in new paid customer creation. With IT budget pressure, customers with a planned migration to Confluent Cloud are likely extending the prep phase and delaying incurring charges as long as possible. There is probably a backlog of free customers ready to switch to paid, which should support paid customer additions over the next year.
Another factor that mitigates concerns around total customer growth is that Confluent has a large pool of existing Kafka users to draw from. In addition to the estimated 75% of the Fortune 500 that have a Kafka installation, over 150,000 organizations total are estimated to be using Kafka. As 36% of the Fortune 500 are already Confluent customers, I think we can assume that Confluent has demonstrated advantages over open source Kafka. These are likely the most discerning customers and historically have received the most focus. With 145,000 (150k – 5k current paying) customers left to convert to Confluent, I think there is adequate runway remaining for total customer growth.
During the Investor Day session in June, Confluent’s Head of Sales discussed how most of the sales and marketing focus to date has been on converting the Fortune 500 and Enterprise customer segments (defined as having >$1B revenue annually) to Confluent. They see an equally large opportunity to monetize the tens of thousands of Kafka users within the Commercial / Mid-market segment (revenue <$1B annually). Over the last 3 years, they have already increased total customers in this segment by 7x and increased $100k ARR customers by 13x.
As another signal around large customer spend in Q2, management noted that the number of $5M+ ARR customers continued to grow, but didn’t provide a number. They also referenced growth in $10M+ ARR customers in the past. During the Investor Day session in June, the head of sales mentioned that Confluent has 9 customers spending $5M+ and 3 with $10+ in ARR.
When I first looked at Confluent, I was surprised to learn that they had customers paying $5M or even $10M a year. Thinking about the importance of the solution for the largest enterprise data companies, it makes sense. As the Confluent platform expands within the enterprise to power the full data distribution backplane (evolves to the “central nervous system”), the importance of the solution rises. It doesn’t require too many $5M-$10M ARR customers to generate $800M in revenue (their current full year target).
Confluent doesn’t provide exact numbers for net expansion, but confirmed that overall NRR for Q2 was still above 130%. The gross retention rate was above 90%. The most impressive metric in my mind, though, is that NRR for just the Cloud service was above 140%. This is quite impressive for a business that has a run rate over $300M. I suspect this will drop below 140% at some point, but is clearly the driver of Cloud’s high annual growth.
Confluent management provided some examples of customer expansion journeys with their largest spenders. Some customers have increased spend by orders of magnitude over a short period of time. One large international bank increased spend by 34x over less than 3 years. An electronics manufacturer grew by 192x in a similar period, but with a smaller base.
It is this opportunity for large customer spend at relatively small scale that gives me confidence in Confluent’s ability to maintain high durable growth over the next few years. Additionally, none of this factors in new products, like stream processing, stream sharing and governance, which management thinks could be as large as the core data streaming product revenue stream over time.
Investment Plan
The primary opportunity for Confluent is to expand their reach across the enterprise data infrastructure fabric to reach the status of “central nervous system”. They have a relatively straightforward glide path in convincing the large installed base of Kafka users to upgrade to Confluent as part of their cloud migration plan. A TCO reduction of 60% and a broader set of capabilities makes this an easy argument for data-intensive enterprise operations (which most are now).
On top of this, Confluent is launching several new capabilities to enable the broader data streaming platform that can each be monetized as add-ons. These include Connectors (monetized now), Stream Governance (monetized now), Stream Processing (early access) and Stream Sharing (just announced). Management has estimated that revenue from Stream Processing could equal that of the core data streaming offering over time.
This expansion motion will in turn drive larger and larger spend by enterprise customers. Confluent already enjoys 147 customers spending more than $1M in ARR. They also reported a number of $5M ARR customers and even a few with more than $10M in ARR. I expect these large customer counts to continue to grow. With a target for less than $800M in revenue this year, Confluent doesn’t require too many customers of this size to make a noticeable impact.
During their Investor Day Presentation, the CFO presented a chart showing the typical growth path for their top 20 Cloud customers. Half of these customers started on the pay-as-you-go (PAYG) plan and eventually reached $1M+ with an ARR multiple of 17x over a 3 year period. On average, they reached $1M+ within just 6 quarters.
In spite of the same demand pressures highlighted by other software infrastructure providers during Q2, Confluent delivered favorable results. Revenue growth exceeded targets and the company increased their projections for the remainder of the year. Profitability showed a strong improvement with beats on EPS and significant increases expected in operating and FCF margin through the remainder of the year. Q4 is still projected to reach operating margin break-even, with FCF margin following the same trajectory.
This performance on the top line was achieved with the 8% headcount reduction in January. To conduct an organizational change like that and maintain revenue targets is pretty impressive. As compared to several other software infrastructure providers, Confluent hasn’t lowered their annual revenue guidance for 2023, which they set back in Q4. To me, this signals that demand for Confluent’s solution remains robust and growth would be significantly higher in a normal spend environment. Having many large customers exceed their committed spend in the quarter further supports the relative uniqueness of Confluent’s performance.
For these reasons, I think Confluent has a favorable set-up going into 2024. We could see revenue growth stabilize in the 30% range and FCF margin inflect to positive. With more focus on profitability by investors, the rapid improvement in profitability measures will be well-received. I have been increasing the allocation to CFLT in my portfolio on Commonstock. It now stands at 10%. I will likely grow this to match other data infrastructure providers like Snowflake and MongoDB over time.

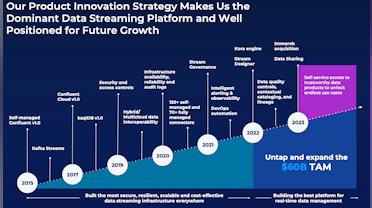
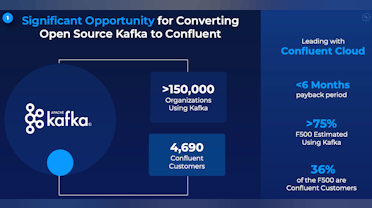

Confluent
Confluent - Pricing
Confluent Cloud - Pricing
@stackinvesting fantastic read as always, thank you Peter!
I'm always amazed how big of a business data services are.
"It is this opportunity for large customer spend at relatively small scale that gives me confidence in Confluent’s ability to maintain high durable growth over the next few years. Additionally, none of this factors in new products, like stream processing, stream sharing and governance, which management thinks could be as large as the core data streaming product revenue stream over time." - I very much agree and would love to see it play through